“Nothing in life is to be feared, it is only to be understood. Now is the time to understand more, so that we may fear less” - Marie Curie, physicist and first woman to win the Nobel Prize
We are used to hearing the term Artificial Intelligence much more frequently these days, but in most non-technical circles, there is an uncomfortable feeling in the air.
The term Artificial Intelligence itself makes a distinction between “real” intelligence that we possess and what machines could have one day -- and “real” is better! Combine this with decades of images from movies and science fiction that paint computers and artificial intelligence as malicious, and it's clear that our relationship to conscious machines is mostly an uncomfortable one. As the father of Artificial Intelligence Marvin Minsky said, “If we're lucky, they might decide to keep us as pets”.
There are scores of experts writing about how we should view the explosion of AI all around us. I approach this topic from the perspective of a parent and educator, trying to understand what we should teach our children so they are not afraid of the world they will live in a decade from now.
Marie Curie says it most succinctly, but I want to provide some more texture here that will hopefully leave you with a clear message for your children!
What is going on today? And why now?
Computers have been getting faster and faster. They have begun to do tasks that are more like the messy, ambiguous things we humans do. For instance, we have made huge progress in the past few years in improving computer vision and object recognition.
This has long been a problem for computers. Although it may seem simple to recognize a dog (and humans can do this at a young age) -- to identify it correctly requires being able to consider both pugs and golden retrievers under the same general category -- and to recognize them even when they are upside down, have their heads buried, are hiding under a blanket or trapped in fog, and to be able to do so in all kinds of light conditions. Computer software would also have to be able to distinguish between a dog and a cat, or a dog and a wolf. There's a lot going on in the object-recognition process -- and programmers have been working on it for a long time.
In 1966 Marvin Minsky, tasked an undergraduate, Gerald Sussman, with building a device that could recognize objects using a computer and a TV. Object recognition is what you do when you recognize that the white blur on a road is a plastic bag flying, and not a deer in sunlight. Mr. Sussman did not fulfill his assignment (although he eventually became a prominent researcher) and the problem remain unsolved for decades -- until now.
Things really started to become exciting in 2007 when Fei-Fei Li, the head of the Stanford AI Lab launched the ImageNet project with a billion downloaded images from the internet. She used crowdsourcing technology like the Amazon Mechanical Turk platform to label these images. This huge amount of labelled data was the perfect match for a specific type of machine learning software called a convolutional neural network, pioneered by Geoffrey Hinton, Kunihiko Fukushima, and Yann LeCun in the 70’s. See this inspiring interview by Geoffrey Hinton talking about how much grit it took to continue working on this approach over multiple decades.
In 2012, Fei Fei announced that Geoffrey Hinton and his students Alex Krizhevsky, Ilya Sutskever, had developed an algorithm that identified objects with almost twice the accuracy of their nearest competitor.
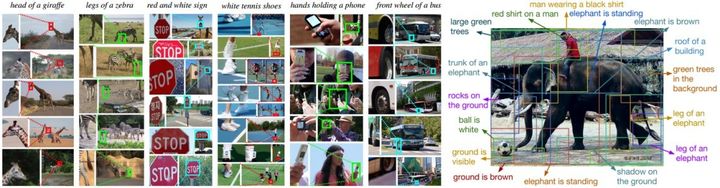
Image Source: Andrej Karpathy, Research Scientist at OpenAI working on Deep Learning in Computer Vision
So why did it take 46 years to solve this problem? Geoffrey Hinton explains that there were a few key factors:
- Our labeled datasets were thousands of times too small.
- Our computers were millions of times too slow.
- Our algorithms weren’t as smart.
So here we are today. The culmination of 50 years of research leading to this:
Deep Learning (a sub-form of Artificial Intelligence) =
Lots of training data + Parallel Computation + Scalable, smart algorithms
Computers are going to continue to get faster and more powerful, training data sets will get larger, the tooling around them will get more accessible and more and more tasks we previously understood to be too messy for anyone but humans to perform will be tackled by computers.
Which presents us with the question at the heart of our sci-fi fears: What will be left for us to do? What should we teach our children to prepare for?
History provides some answers.
In 1900, 40% of jobs in the United States were in agriculture, and a significant percentage of all jobs required hard physical labor. Physical strength and stamina were desired job skills. A century later, because of science, automation and better farming techniques, only 2% of jobs in the United States were in agriculture. A new field of “precision agriculture” has emerged, and sectors such as health care, finance, information technology, consumer electronics, hospitality, leisure, and entertainment employ significantly more workers (Autor, 2014). The four C’s - critical thinking, creativity, communication and collaboration have become the desired job skills.
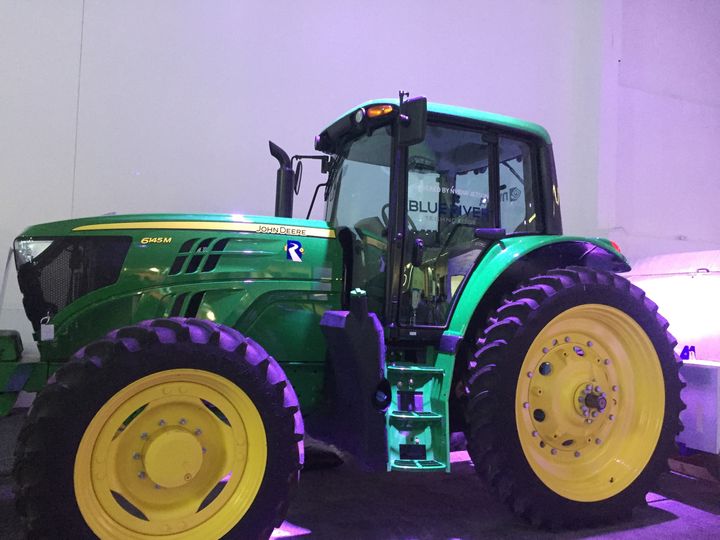
John Deere tractor powered by Blue River Technology at the NVIDIA GTC
Or in another example, the introduction of the automobile completely eliminated the jobs of carriage makers, but resulted in a whole lot of new jobs for auto-body makers. As car prices dropped, the demand for cars grew, and there were more jobs for auto-body makers. In 1895 there were only four cars officially registered in the U.S. Little more than 20 years later in 1916, 3.6 million were registered causing numerous entrepreneurs and inventors (GM, Ford, Olds Motor Company, Cadillac, Chevrolet) to plunge into the auto-making business (Bessen 2016).
The development of Spinning Mule technology in the 18th century also resulted in the loss of a particular type of job, but increase in another. Without the Spinning Mule it would take a worker over 50,000 hours to spin 100lbs of cotton manually. However, by the latter part of the 18th century it took only 300 hours to spin the same amount with the Spinning Mule and only 135 hours via a self-acting mule. By the 19th century, almost 98% of weaving was automated, but the number of weaving jobs actually increased (Bessen 2015). The reason for this enigmatic result was that automation drove the price of cloth down, resulting in cloth being used in all sorts of ways, increasing demand and overall job growth in the weaving industry.
In the field of financial services, a similar dynamic demand response was seen when the number of ATMs increased from 100,000 to 400,000 between 1995 and 2010. As the cost of operating branches decreased, it enabled banks to open more branches, increasing in the number of jobs for bank tellers. And interestingly, the role of the bank tellers changed to focus more on the relationship with the customer (Bessen 2016). Their ability to market and their interpersonal skills in dealing with customers became more important than the skill of counting out notes.
Self-service kiosks have similarly intriguing effects on customer behavior. For instance in some fast food restaurants customers spend more money on add-ons, drinks etc as they do not feel guilty asking the computer, and the technology doesn’t forget to ask!
However self-service kiosks are not as successful in grocery stores as they shift the work to the customer and require the customer to do a type of work that they may not expect to do, want to do or even be able to do.
In the end, we are social creatures and social interactions make us happier. Technology can help smooth out the rough edges of these types of interactions in unpredictable ways, but cannot fully replace them. Thus complete automation is unlikely. Looking at historic examples, what is more likely is an increase in partial automation occurring alongside the opening up of new jobs and roles for humans. We will have to find new ways to work alongside machines, and retool our skill-sets regularly.
In the words of the Red Queen in Alice in Wonderland, “…it takes all the running you can do, to keep in the same place.”
But lifelong learning need not seem burdensome. Video game playing statistics show that we like novelty, challenges, and developing new skills and competencies -- when they are presented like video games! What this means for non-game learning environments is that it should be easy to get started, feedback should be personalized and rapid, failure should be private and success should be public, the reason for acquiring the skills should be powerful and there should be meaningful social and physiological elements to the learning experience. When the stars align, self-directed learning is fun. And this is what we need today.
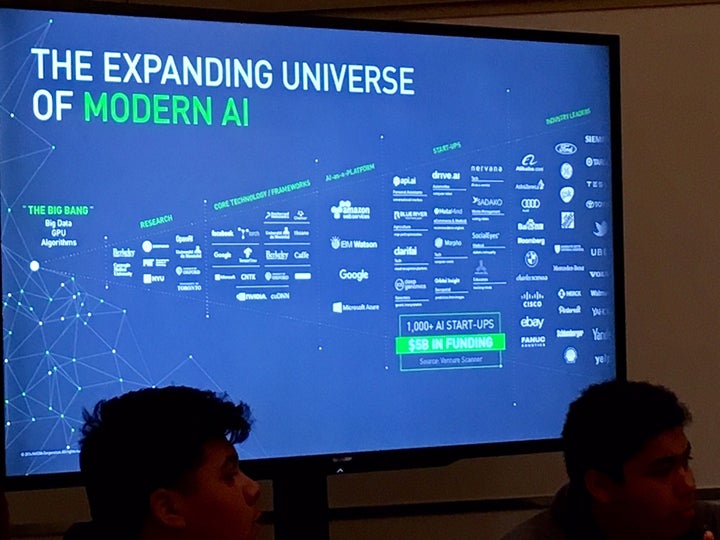
We also need to model lifelong curiosity and learning for our children, and we need to take tangible steps towards understanding the key elements of Artificial Intelligence. Even basic familiarity with these elements will give a sense of empowerment and confidence. And more importantly our children need to embrace the new normal of lifelong learning. Programmers do this all the time to stay up to date. Now other disciplines need to adopt this perspective, especially jobs in trucking and possibly even coal mining.
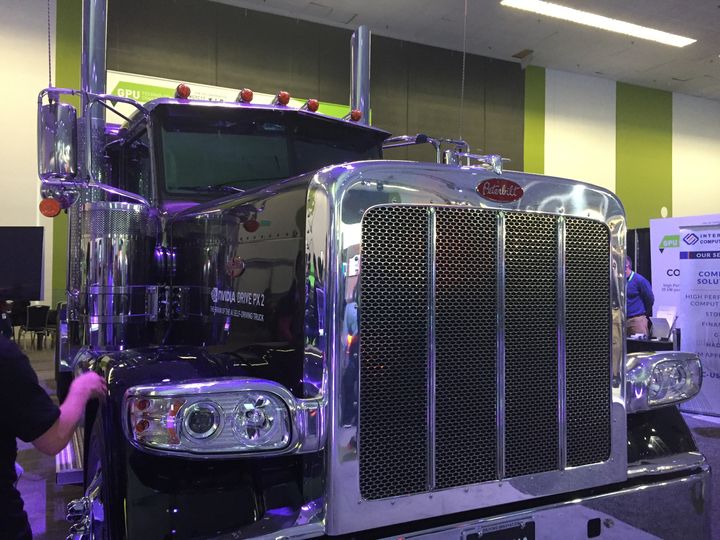
PACCAR truck powered by NVIDIA DRIVE PX 2 technology at the NVIDIA 2017 GTC
NVIDIA is one of the leaders in Artificial Intelligence -- specifically, a subset called Deep Learning, where machines learn based on algorithms as well as their own experiences/interactions with large data sets. Recently they did something unusual. They partnered with a STEM education nonprofit - Iridescent - and opened up their signature developer conference - GTC - to 200 local high school students to work closely with NVIDIA experts and learn more about parallel processing, neural networks and self-driving cars.
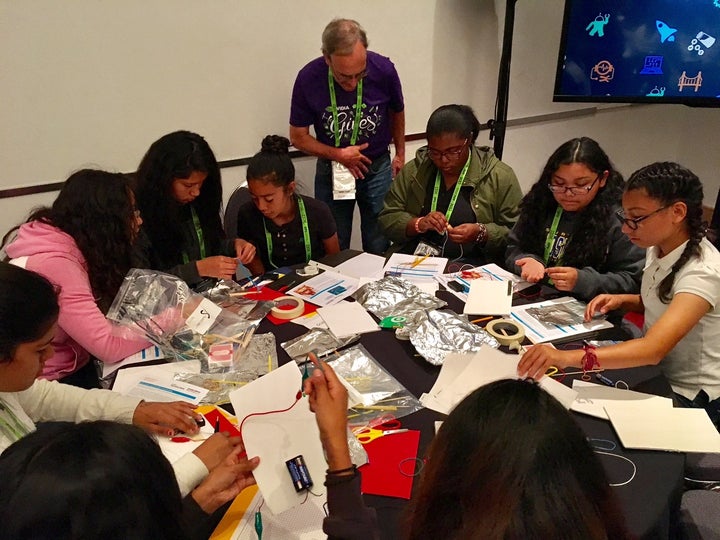
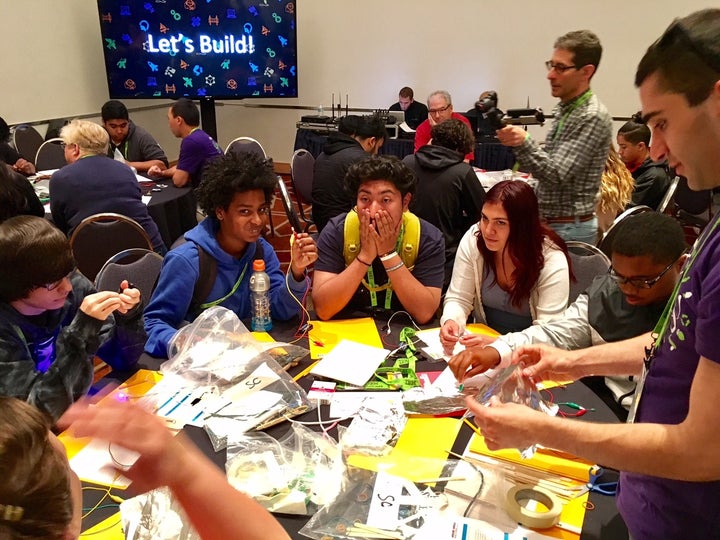
Students were faced with three different challenges - to create a physical network that classifies different types of information by sending data through different nodes; to build a system of circuits to simulate sensors and rapid decision making similar to that in a self-driving car; and to work in teams to create a physical parallel processing system that could sort objects quickly and accurately using multiple channels. All design challenges used very simple materials, but brought to life some of the core concepts underlying parallel computation, neural networks and autonomous vehicles.
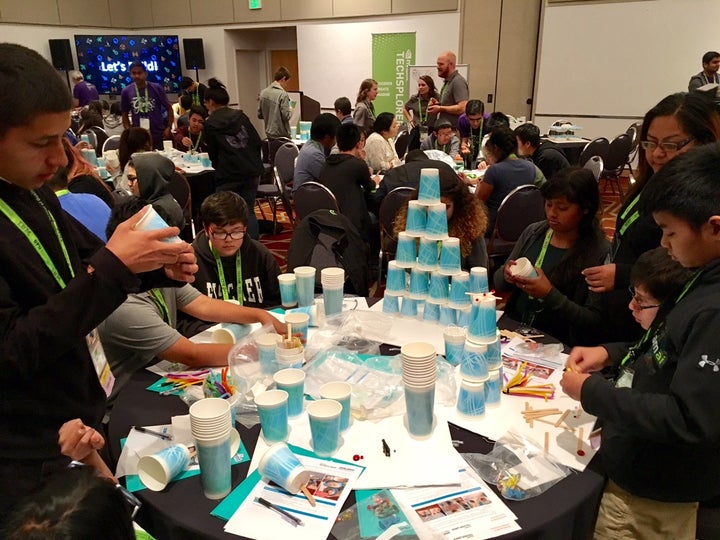
These design challenges are a great way to engage children all over the world and introduce the amazing world of Artificial Intelligence to them, and most importantly, show them how they have a role to play in making it even more intelligent.
"Problem-solving is like when a pattern won't work because something new happened, and you need to find out why to adjust the pattern so it works again, but you will need to do this over and over again and this is the learning process for AI." - Luis, High School Student