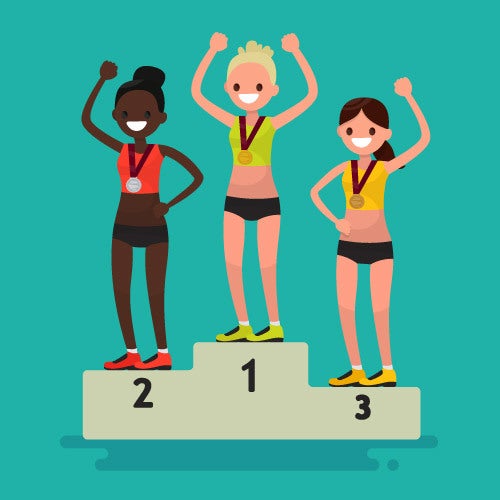
As anyone who reads my blogs is aware, I’m a big fan of the ESSA evidence standards. Yet there are many questions about the specific meaning of the definitions of strength of evidence for given programs. “Strong” is pretty clear: at least one study that used a randomized design and found a significant positive effect. “Moderate” requires at least one study that used a quasi-experimental design and found significant positive effects. There are important technical questions with these, but the basic intention is clear.
Not so with the third category, “promising.” It sounds clear enough: At least one correlational study with significantly positive effects, controlling for pretests or other variables. Yet what does this mean in practice?
The biggest problem is that correlation does not imply causation. Imagine, for example, that a study found a significant correlation between the numbers of iPads in schools and student achievement. Does this imply that more iPads cause more learning? Or could wealthier schools happen to have more iPads (and children in wealthy families have many opportunities to learn that have nothing to do with their schools buying more iPads)? The ESSA definitions do require controlling for other variables, but correlational studies lend themselves to error when they try to control for big differences.
Another problem is that a correlational study may not specify how much of a given resource is needed to show an effect. In the case of the iPad study, did positive effects depend on one iPad per class, or thirty (one per student)? It’s not at all clear.
Despite these problems, the law clearly defines “promising” as requiring correlational studies, and as law-abiding citizens, we must obey. But the “promising” category also allows for some additional categories of studies that can fill some important gaps that otherwise lurk in the ESSA evidence standards.
The most important category involves studies in which schools or teachers (not individual students) were randomly assigned to experimental or control groups. Current statistical norms require that such studies use multilevel analyses, such as Hierarchical Linear Modeling (HLM). In essence, these are analyses at the cluster level (school or teacher), not the student level. The What Works Clearinghouse (WWC) requires use of statistics like HLM in clustered designs.
The problem is that it takes a lot of schools or teachers to have enough power to find significant effects. As a result, many otherwise excellent studies fail to find significant differences, and are not counted as meeting any standard in the WWC.
The Technical Working Group (TWG) that set the standards for our Evidence for ESSA website suggested a solution to this problem. Cluster randomized studies that fail to find significant effects are re-analyzed at the student level. If the student-level outcome is significantly positive, the program is rated as “promising” under ESSA. Note that all experiments are also correlational studies (just using a variable with only two possible values, experimental or control), and experiments in education almost always control for pretests and other factors, so our procedure meets the ESSA evidence standards’ definition for “promising.”
Another situation in which “promising” is used for “just-missed” experiments is in the case of quasi-experiments. Like randomized experiments, these should be analyzed at the cluster level if treatment was at the school or classroom level. So if a quasi-experiment did not find significantly positive outcomes at the cluster level but did find significant positive effects at the student level, we include it as “promising.”
These procedures are important for the ESSA standards, but they are also useful for programs that are not able to recruit a large enough sample of schools or teachers to do randomized or quasi-experimental studies. For example, imagine that a researcher evaluating a school-wide math program for tenth graders could only afford to recruit and serve 10 schools. She might deliberately use a design in which the 10 schools are randomly assigned to use the innovative math program (n=5) or serve as a control group (n=5). A cluster randomized experiment with only 10 clusters is extremely unlikely to find a significant positive effect at the school level, but with perhaps 1000 students per condition, would be very likely to find a significant effect at the student level, if the program is in fact effective. In this circumstance, the program could be rated, using our standard, as “promising,” an outcome true to the ordinary meaning of the word: not proven, but worth further investigation and investment.
Using the “promising” category in this way may encourage smaller-scale, less well funded researchers to get into the evidence game, albeit at a lower rung on the ladder. But it is not good policy to set such high standards that few programs will qualify. Defining “promising” as we have in Evidence for ESSA does not promise anyone the Promised Land, but it broadens the number of programs schools may select, knowing that they must give up a degree of certainty in exchange for a broader selection of programs.
This blog is sponsored by the Laura and John Arnold Foundation